Reading the economy: short-term forecasts from ExportPlanning
Using ARIMAs allows us to have a robust and reliable economic scenario
Published by Simone Zambelli. .
Conjuncture Forecast Uncertainty Internationalisation tools
Log in to use the pretty print function and embed function.
Aren't you signed up yet?
signup!
In situations of great uncertainty, economic analyses assume increasing importance, that is analyses that can best use available information to measure economic changes that have occurred in the nearer past.
A case of particular interest are forecasts that deal with foreign trade dynamics. The importance of being able to have a timely update of the main trade statistics is in fact twofold: on the one hand, they represent an objective market benchmark for exporting companies in order to assess their performance towards the main geographies served; on the other hand, only the constant monitoring of these data allows for the pursuit of destinations that are more resilient to a slowing economic cycle such as the current one.
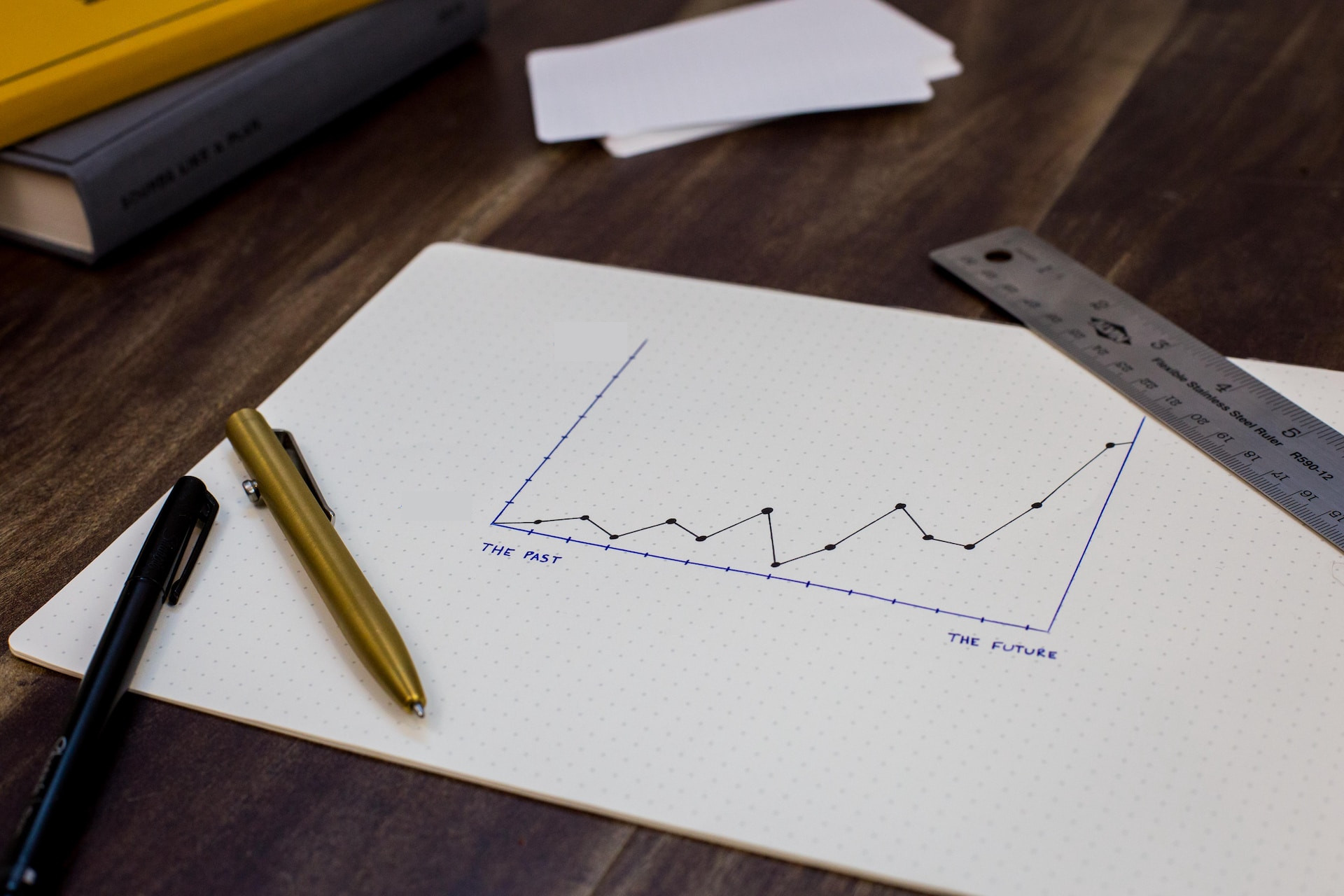
However, having as much information as possible, in order to enjoy the most up-to-date data in a timely manner, is not trivial and requires special attention. Indeed, not all countries make foreign trade statistics available with the same timing.
For example, foreign trade data from European countries are made available by Eurostat through the Comext database, usually with a delay of about two months (as of today we have those for January 2023). However, there is a different publication timing for trade flows to Intra-EU and Extra-EU countries: the former are in fact declared only by a small sample of countries, while the latter are declared by all European countries, causing a one-month mismatch between the two of declarations.
A first way to overcome this difficulty would be to consider as the last period of analysis that in which there is full availability of information for all countries in the sample. However, this way of proceeding would cause important information to be lost, which may be crucial to a correct economic reading.
It can be understood, therefore, how there is a trade-off between sample of countries to be considered and updating of the same estimate in the analysis of foreign trade information. If one considers only those countries that are faster in publishing foreign trade data, it is possible to construct a very up-to-date measure of international trade dynamics, but with less representativeness of the population of countries. Conversely, if one wants to have a sample that is close to the entire population, one must necessarily also consider those countries that lag behind in their reporting.
How does ExportPlanning overcome such difficulties?
There are a number of stochastic methods that can be applied to get around this problem by bringing the data forward by a few months and "evening out" the countries in the sample that have not yet reported.
Autoregressive Integrated Moving Average (ARIMA) models allow for very reliable short-term forecasting. These econometric models are based on the concept that the future behavior of a certain phenomenon is a function of its past behavior. Thus the forecasting task becomes, in essence, a careful analysis of the past plus an assumption that the same patterns and relationships will hold in the future. In order to apply this model, certain steps are required, such as verifying that the time series is stationary (the statistical characteristics of the time series do not change over time) and identifying parameters of autoregressive (AR), integration or differentiation (I), and moving average (MA) types.
ExportPlanning's ARIMA models use purpose-built supervised machine learning algorithms in order to determine the right components to be able to apply the most robust and correct estimation model to the data.
The time series under consideration are estimated at a detailed 6-digit Harmonized System product code and individual declarant-partner flow level, so as to isolate individual exchanges effectively.
The advantage of having these models is that they can estimate missing country statements, as well as robustly estimate time series by bringing them forward a few months, so that quarterly trends can be closed well in advance.
A practical example on European data
A practical example of the effectiveness of this methodology is the application of ARIMAs to the trade flows of European countries.
The graph below shows the rates of change in European exports, where the focus is on the fourth quarter of 2022. The gray bars represent the quarterly rates of change of European exports calculated based on totally historical data updated to December 2022; the green bars, on the other hand, show the quarterly rate of change derived based on data updated to October 2022 for Intra-EU and November 2022 for Extra-EU, then supplemented to December through the use of ARIMA forecast models.
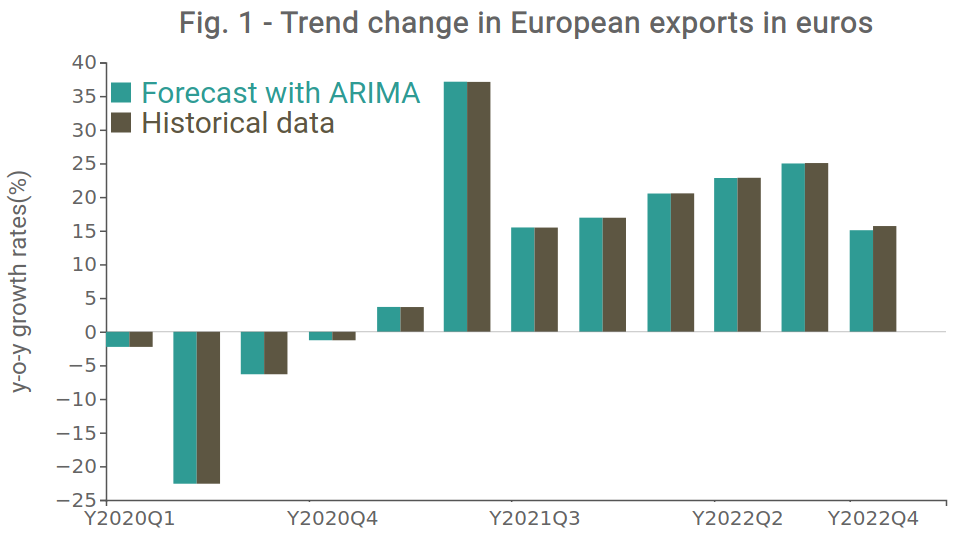
Source: ExportPlanning.
As we can see from the graph, the strong proximity of the two results for the fourth quarter of 2022 is evident, with very little difference, reflecting the effectiveness of the estimate made more than two months earlier. This methodology allowed us to pre-estimate the year-end of European exports in advance of the publication of the historical figure by Eurostat.
Conclusions
The analysis just conducted demonstrated the importance of missing data estimation and short-term time series forecasting using ARIMAs.
Through this methodology, the robustness of foreign trade estimates from all ExportPlanning databases is ensured. This is particularly important for timely economic readings and can help companies that want to export gain an information advantage over their main competitors.